# Accelerating MRI Imaging with AI: Facebook and NYU's Innovative Approach
Written on
Chapter 1: Introduction to FastMRI Technology
The quest to expedite magnetic resonance imaging (MRI) has been a long-standing objective in the medical field. Recently, Facebook's Artificial Intelligence Research group (FAIR) partnered with New York University’s Medical School to explore the use of AI algorithms in enhancing MRI efficiency. Their collaborative efforts aim to reduce the MRI duration from approximately an hour to just 15 minutes. The initial findings from their research, named FastMRI, suggest that the resulting scans maintain quality comparable to traditional MRI methods.
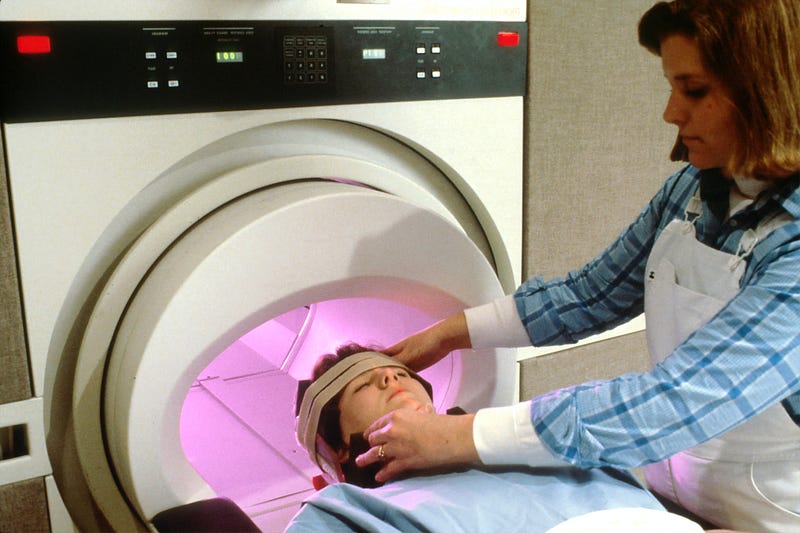
Chapter 2: The Study and Its Findings
In a comprehensive study involving NYU researchers, conventional MRI scans of the knees of 108 patients were analyzed. The team extracted a portion of the raw data and utilized AI to generate new MRI images based on this limited dataset. Six radiologists assessed all the scans and provided clinical recommendations. The results demonstrated that the radiologists issued similar recommendations for both the original and FastMRI scans, with a preference for the latter in terms of image quality. Notably, only one radiologist could reliably differentiate between the two imaging techniques, indicating a potential for FastMRI’s acceptance within the medical community.
Chapter 3: Understanding MRI Mechanics
MRI machines, typically donut-shaped, produce images of soft tissues in the body by generating a magnetic field and radio frequency pulses. The intricate process can take over an hour, which impacts the number of patients that can be scanned daily and contributes to higher costs. FastMRI aims to streamline this by eliminating about 75% of the data points usually collected, allowing AI to fill in the missing information. This approach not only accelerates the imaging process but also enhances clarity, which is why radiologists favored FastMRI.
Section 3.1: Validating FastMRI Technology
The primary goal of the study was to ascertain whether FastMRI images could serve as substitutes for traditional MRI scans. Concerns about potential artifacts or omissions in the images were addressed, with findings indicating that FastMRI did not introduce such issues. Nafissa Yakubova, a researcher at FAIR, noted that their deep learning models were trained on existing MRI knowledge to ensure accuracy.
Subsection 3.1.1: Future Directions for FastMRI
Despite promising results, the study had limitations, focusing solely on one type of MRI machine and the soft tissue of the knee. Dr. Dan Sodickson from NYU Langone Health is collaborating with various institutions to conduct multisite studies to further validate FastMRI technology. This open-source technology allows for widespread access, and researchers are looking to extend its application to other body regions.
Chapter 4: Broader Implications of AI in MRI
While other institutions have previously sought to enhance MRI imaging speed, Facebook's involvement raises questions about their motivations. Rather than pursuing profit, Yakubova explained that FAIR aims to gain valuable insights from the complexities of MRI imaging. The rigorous standards required in medical imaging present a unique challenge for AI, with the potential to inform future advancements in computer vision.
In summary, the integration of AI into MRI imaging not only promises to make this critical healthcare tool more accessible but also offers significant learning opportunities for advancing artificial intelligence technologies.