The Future of Artificial Intelligence: A Long Road Ahead
Written on
Chapter 1: The Dartmouth Conference and Its Legacy
In the summer of 1956, a distinguished group of researchers converged at Dartmouth College. Spearheaded by notable figures such as John McCarthy, Marvin Minsky, Nathaniel Rochester, and Claude Shannon, their objective was both ambitious and groundbreaking: to investigate and articulate the possibilities of machines emulating human intelligence. The researchers were optimistic, believing they could delineate every aspect of learning and intelligence so precisely that machines could be programmed to mimic these processes.
With a modest grant of $7,500 from the Rockefeller Foundation, the project was initially intended to span just eight weeks. Although they did not achieve their ambitious goal of fully simulating human intelligence during this timeframe, they did coin the term "Artificial Intelligence," effectively laying the groundwork for a new field of scientific inquiry.
Around the same period, across the Atlantic in Germany, Prof. Dr. Karl Steinbuch was making significant contributions to computer science and artificial intelligence. Now regarded as a pioneer in AI, Steinbuch's innovations were foundational to the discipline. He was instrumental in establishing the term “Informatik” (informatics), which became synonymous with computer science. His contributions extended beyond theory; he constructed the first large-scale computer for commercial purposes in Germany, commissioned by the mail-order company “Quelle.”
Steinbuch distinguished himself by concentrating early on AI and machine learning. He articulated essential principles of AI systems that remain relevant today and developed some of the first self-learning systems. Despite the primitive technology of his era, he created machines capable of letter recognition using self-learning principles.
These early forays into pattern recognition and machine learning were revolutionary, illustrating that computers could be programmed to adapt and enhance their capabilities through experience—an essential tenet of contemporary AI.
Wherever possible, I highly recommend reading the works of Prof. Dr. Karl Steinbuch. His ability to foresee developments in IT as early as the 1960s is remarkable. He had to utilize the language and concepts available during his time, yet his predictions about technological innovations and their societal impacts—both positive and negative—were astonishingly accurate. He even foresaw the emergence of smartphones nearly four decades before they became commonplace.
Had one read his books back then and acted on his insights, it would have been an unparalleled investment opportunity. Steinbuch also elaborated on why it may take several decades, or even centuries, to create truly intelligent AI systems that can replicate human capabilities.
He was not skeptical about the potential of AI; rather, he was optimistic that humans could eventually replicate everything that nature has produced, including the intricacies of the human brain. However, he noted several limitations:
The human brain remains a mystery, both then and now. Steinbuch suggested that it might even be inherently impossible for us to fully comprehend our own cognitive processes. More critically, the human brain is an incredibly intricate organ, comprised of hundreds of billions of neurons.
The challenge lies in the fact that constructing a computer with a hundred billion circuits would not bring us close to replicating brain function. This is not solely due to our lack of understanding of the brain's workings; it also pertains to computing power. While computer circuits operate using binary code—ones and zeros—the neurons in the human brain can transmit signals in numerous ways and intensities.
It doesn't take a genius to realize that this exponentially amplifies the human brain's potential compared to a computer with an equivalent number of circuits.
Thus, while we still lack a complete understanding of the human brain, our current computational capabilities are far from matching its complexity. Steinbuch, who predicted a form of Moore's law before it was articulated by Gordon Moore, anticipated that it would take at least another century, and possibly much longer, to develop computers that can rival all facets of human intelligence.
Certainly, what we label as AI today can perform remarkable tasks and significantly enhance human abilities. However, the gap between true AI and our current capabilities is likely as wide as it was for scientists in 1956 during the Dartmouth Project.
Although technology has advanced tremendously, and some tools have become invaluable in everyday life, we are still not in the realm of "intelligence."
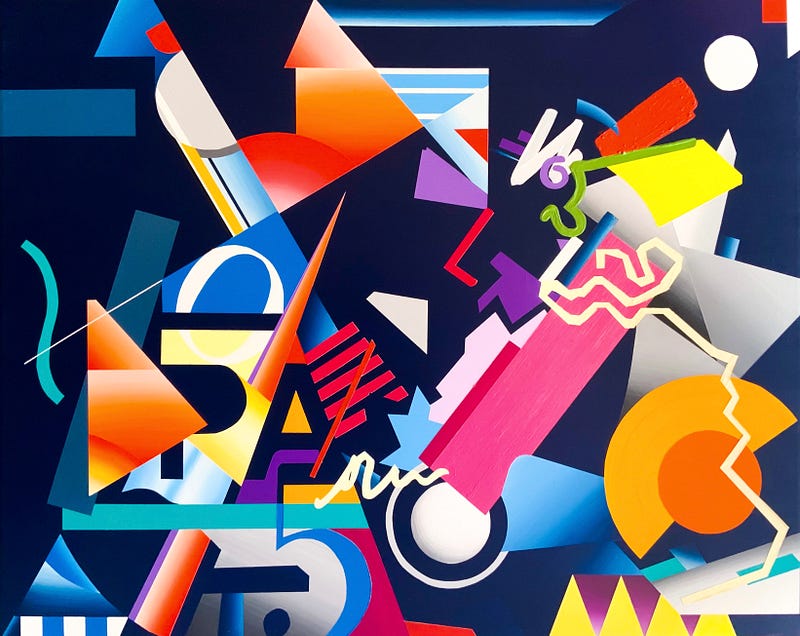
Chapter 2: Exploring Modern AI Perspectives
The first video offers insights into the notion that artificial intelligence, as commonly understood, may not exist in the way we envision it today. In "There is no such thing as Artificial Intelligence," Luc Julia discusses the limitations and misconceptions surrounding AI.
The second video, "There's No Such Thing as Artificial Intelligence" by John Hornsby, further delves into the complexities and challenges of developing true AI.