Innovative Approaches in Credit Risk Modeling Using Data Science
Written on
Chapter 1: Understanding Credit Risk in Financial Lending
When financial institutions extend loans, they inevitably encounter risks. To mitigate these risks, lenders aim to provide loans only to those clients they believe will reliably repay them.
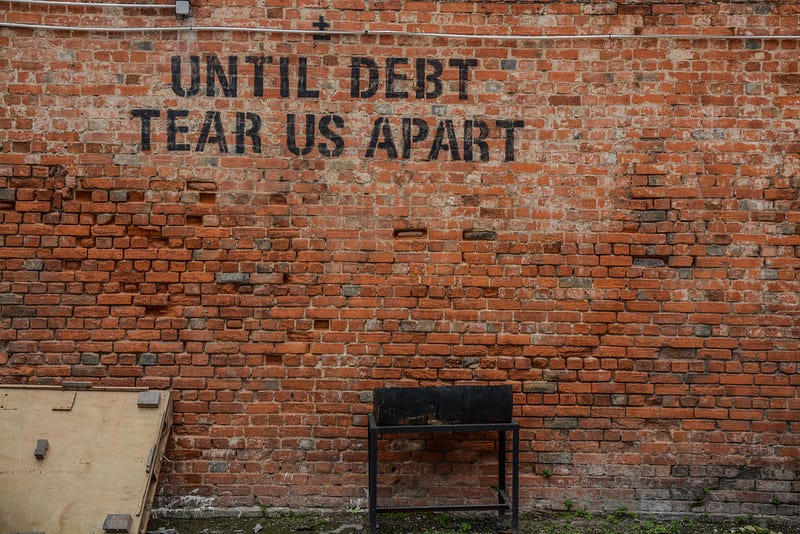
Historical Context of Credit Scoring
The foundation of contemporary credit scoring was established by Ronald A. Fisher in 1936, who introduced a statistical method known as discriminant analysis. This technique was utilized to differentiate between groups based on quantifiable attributes. In 1941, Durand adapted this method to assess the viability of loans, leading to the practice known as "credit scoring." This process assigns numerical scores to loan applicants, reflecting their likelihood of timely repayment. Banks leverage these scores to implement risk-based pricing, often resulting in higher interest rates for borrowers with lower scores.
Limitations of Traditional Credit Assessment
One of the main drawbacks of conventional credit evaluation methods is their rigidity. Traditional systems rely heavily on established rules, which can disadvantage applicants with imperfect credit histories. This raises questions: How can an individual without prior banking experience secure their first loan? What about entrepreneurs seeking funding for startups? These complexities are often overlooked by traditional credit models, which tend to favor individuals with established repayment histories.
Current assessments reveal several critical weaknesses in traditional credit scoring systems:
- Reliance solely on historical data
- Evaluation of variables in isolation
- Human biases affecting risk assessment parameters
- Limited capacity to understand only linear relationships
- Lack of nuance in rule-based logic
- Excessive dependence on structured data
Data Science's Role in Credit Scoring
The integration of data science into financial services can significantly enhance the credit scoring process. The initial step involves comprehensively understanding the data and conducting feature engineering to establish a baseline model. Following this, machine learning algorithms can be employed to refine the credit scoring methodology.
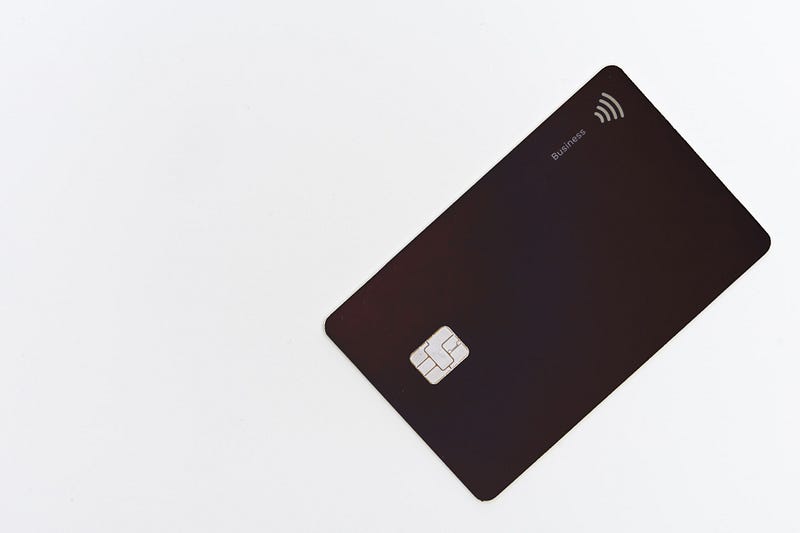
Section 1.1: Broadening the Dataset Analysis
Identifying new risk indicators is crucial for responsive credit scoring. Unlike traditional methods, data science can capture nuances that are often missed by human evaluators and scorecard systems. By utilizing a self-learning machine learning framework, financial institutions can achieve more accurate and comprehensive evaluations of customer profiles. Feature engineering plays a pivotal role in developing a robust baseline model for credit scoring applications.
Section 1.2: Enhancing Predictive Accuracy
Conventional credit scoring models typically analyze historical data linearly to predict future creditworthiness. In contrast, self-learning machine learning systems leverage both past and current data to enhance their predictive capabilities. Techniques aimed at preventing overfitting and fine-tuning hyper-parameters can be employed, enabling the analysis of extensive datasets. This approach facilitates the discovery of connections between disparate variables, offering deeper insights into borrowers' profiles. Validation datasets, evaluated with ROC-AUC curve scores, should be employed to continuously enhance scoring accuracy.
Chapter 2: Evolving the Credit Scoring Process
Recalibration and Adaptability
Traditional scoring models often struggle with the integration of new parameters, which can slow down processes and complicate evaluations. In contrast, machine learning algorithms are inherently more adaptable, allowing for automatic updates and improvements over time. This adaptability is achieved through monitoring overfitting with cross-validation, which also aids in model selection.
Cost-Effectiveness of Machine Learning Models
While some may view machine learning systems as expensive to implement, they can prove to be more cost-effective over time. Once established, these models can be reused across various credit applications. Unlike traditional scorecard systems, which often charge per user, machine learning solutions offer a customizable and continuously evolving framework that meets diverse credit scoring needs. For example, ensemble machine learning models can deliver flexible and accurate assessments of credit eligibility and borrower ranking, ultimately minimizing the risk of issuing "bad" loans.
Conclusion: The Future of Credit Risk Scoring
The application of machine learning and data science in credit risk assessment presents a scalable solution for modern financial challenges. Data engineers can enhance platform capacity, while data scientists can refine models to improve credit scores across the board. A well-implemented data science solution not only increases credit accessibility for a broader customer base but also enables financial institutions to reach underserved populations.
This video explores credit risk analysis through data science and machine learning, complete with source code.
Discover how to model credit risk using Python in this informative video series focusing on data analysis techniques.
Subscribe to our Acing AI newsletter for more insights into data science and machine learning!