The Rise of LLaVa-1.5: A Breakthrough for Open-Source AI
Written on
Chapter 1: Understanding the Multimodal Shift
The ongoing competition between open-source and proprietary AI models has often led to the same conclusion: open-source platforms appear promising but frequently fall short of practicality. However, recent developments may signify a turning point.
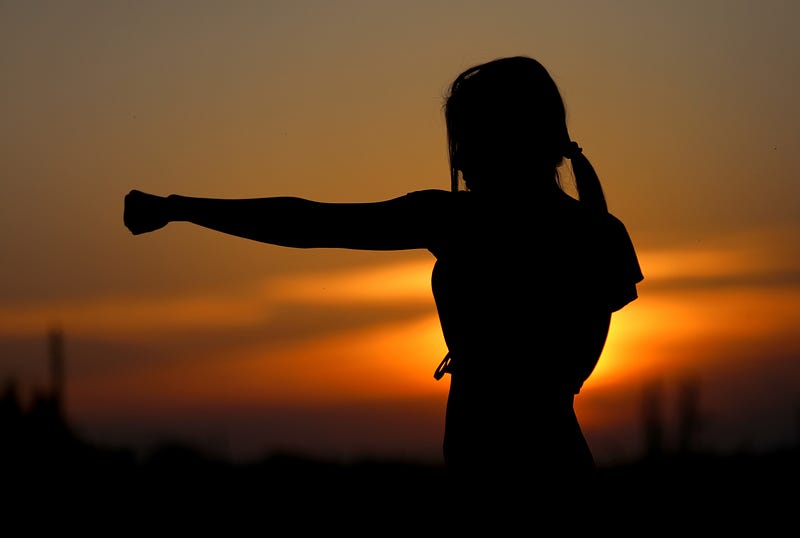
Microsoft, in collaboration with the Universities of Wisconsin-Madison and Columbia, has introduced LLaVa-1.5, an enhanced version of its groundbreaking LLaVa model. This model stands out as one of the first genuinely effective Large Multimodal Models (LMMs) and boasts remarkable performance, especially considering its size is significantly smaller—by hundreds of times—than leading models like OpenAI's GPT-4 Vision.
The newly published research not only sheds light on the construction of advanced multimodal models but also challenges widespread assumptions about the viability of open-source solutions, including my own previous skepticism.
This article was initially featured in my free weekly newsletter, TheTechOasis. If you wish to keep pace with the rapidly evolving AI landscape and feel empowered to take action—or at least prepare for the future—consider subscribing below to become a leader in the AI domain:
Subscribe | TheTechOasis
The newsletter to stay ahead of the curve in AI
thetechoasis.beehiiv.com
The Concept of Multimodality Explored
In discussions surrounding AI, the term "multimodality" is frequently used, but its meaning can often be obscured. Essentially, multimodality refers to a model’s ability to handle at least two different types of input data (such as text, images, sounds, etc.).
To clarify, while a system can appear to be multimodal by integrating various models (like a speech-to-text model with a language model), this does not necessarily mean that the individual models are inherently multimodal. For instance, ChatGPT's latest GPT-4V version can process images and text, but it does not inherently merge these modalities at the model level.
In true multimodality, different input types share a common embedding space, allowing the model to interpret and relate various modalities similarly to how humans do.
The pivotal question arises: how did the researchers achieve this with LLaVa-1.5?
Grafting: A Novel Approach to Multimodality
There are multiple strategies to achieve multimodality:
- Tool/Model-Based Methods: Combining different models or tools to handle multiple inputs while the underlying models remain unimodal.
- Grafting: Utilizing pre-trained image encoders and language models, projecting the encoder's vector into the language model's latent space.
- Generalist Systems: Training both image encoders and language models from scratch in a shared embedding space.
LLaVa-1.5 employs the grafting technique, which is advantageous because the weights of the image encoder and the language model remain static while only the projection matrix is trained.
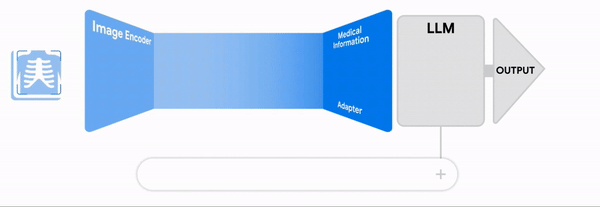
This method facilitates a cost-effective training process, allowing for substantial performance gains without the need for extensive computational resources.
Impressive Results from LLaVa-1.5
The performance metrics for LLaVa-1.5, particularly the 13B model, are striking. Out of twelve prominent multimodal benchmarks, LLaVa-1.5 achieved the state-of-the-art (SOTA) status in eleven, outperforming many other open-source models significantly.
While it’s clear that LLaVa-1.5 does not match GPT-4V in absolute terms, it excels relative to its size and resource requirements, often needing 2,500 times less data for pre-training and 75 times less for fine-tuning.
Remarkably, this model was trained in just one day using an 8-A100 NVIDIA GPU node over a span of 26 hours, suggesting that open-source solutions are finally becoming more accessible and affordable.
As a result, enterprises can now harness their data to develop open-source models that elevate their competitive edge without relying on third-party APIs. In a financial landscape driven by efficiency, having a viable model is just as critical as having the best one. In this context, LLaVa-1.5 stands out as both effective and sustainable within the open-source LMMs landscape.